Latest Posts
Last edited: 2021-03-15 17:16:45
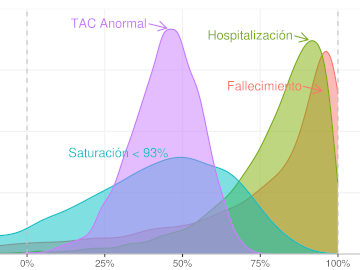
Estimaremos la Eficacia de varias vacunas contra Covid-19 (Pfizer, Moderna, AstraZeneca, y Sputnik), usando el mismo método de estimación para todas, que nos permitirá compararlas mirandolas a través un mismo lente.
Aprovecharemos para evaluar la Eficacia de las dos vacunas Sinopharm ensayadas en Perú, la de los Institutos Beijing y Wuhan, según los datos filtrados a la prensa. Por supuesto, la validez de esos resultados esta condicionada a qué tanto la data filtrada corresponde a la data final y completa del ensayo de las vacunas.
Usaremos el modelo empleado por Pfizer para reportar su ensayo, y también un método más robusto, con un Modelo Bayesiano completo. Ambos modelos son explicados en detalle.
Last edited: 2021-03-14 17:59:01
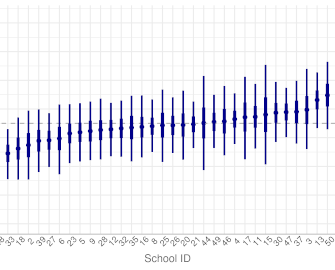
In 1989, Peter Mortimer published "A study of effective junior schools". There he wants to find out how much the students' background influences his/her learning ability. But his main focus is to determine whether individual schools can affect students learning.
We will look at Mortimer's study data, analyzing it as an exercise for practicing multilevel regression using full Bayesian analysis. We will find how much schools make a difference.
Last edited: 2021-03-13 17:16:45
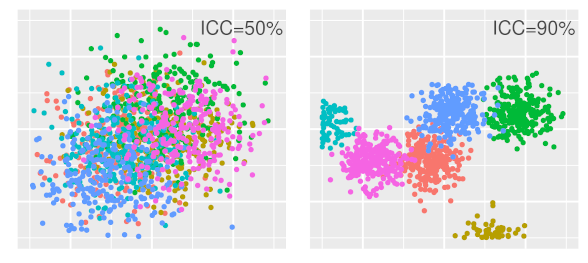
In data analysis, we often want to contrast how certain groups in the data affect an outcome variable. For example, the school's effect on the student's learning, the education on the voter political preferences, or the boss's on employee performance.
When this is the case, doing a separate regression for each group is an analysis option. However, the problem then is that each group's model completely ignores others' observations. Ignoring the general data pattern causes overfitting when there are few observations in a group.
Another alternative is to ignore the groups and do a single regression for all the data. But when the analysis's focus is to compare each group's effect, this path is not feasible.
A multilevel regression incorporates in a single model both the trend within each group and the general pattern in all the observations, enabling us to find out how much each group affects the outcome variable.
Last edited: 2021-04-05 14:59:23
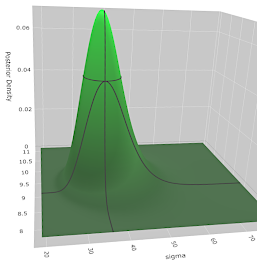
The Bayesian paradigm treats the model’s parameters like random variables whose different values make the model produce data that is more or less likely to come from the same distribution as the observed data.
This post develops the Bayesian inference concepts through their application in a practical example, solving a simple regression model numerically, in a fully Bayesian way. We will match the theoretical concepts with their practical implementation, helping you to grasp their meaning entirely.
Last edited: 2020-12-31 16:16:20
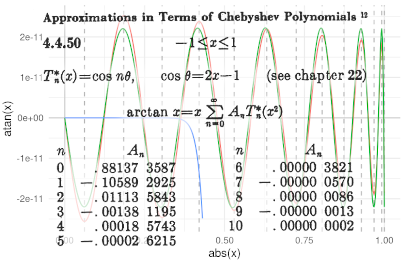
When I was young, I was curious to know how a computer calculates functions such as the cosine, the logarithm, or the tangent's inverse, the so-called elementary transcendental functions.
It turns out that they are calculated with the so-called Chebyshev polynomial approximation, where you get each polynomial coefficient by solving an integral.
In some cases, you need a couple of tens of coefficients, so there are lots of integrals to solve. It is more complicated when the function you want to approximate appears in those integrals, because you don't have how to calculate that function yet, and that's precisely why you want to have the approximation!
With patience and a little luck, I could analytically solve the approximation to some of these functions; and here I will tell you how I did it.
Last edited:
Last edited:
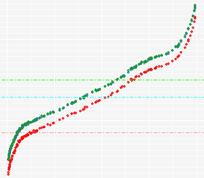
Last edited:
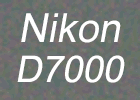
Last edited:
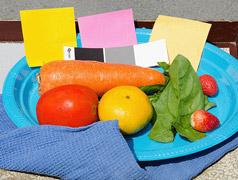
Last edited:
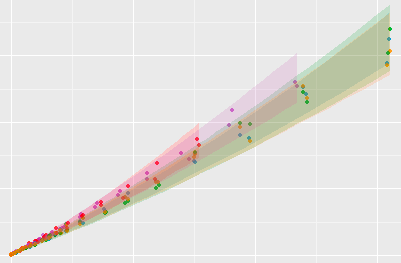
Last edited:
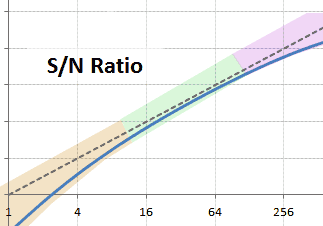
Last edited:
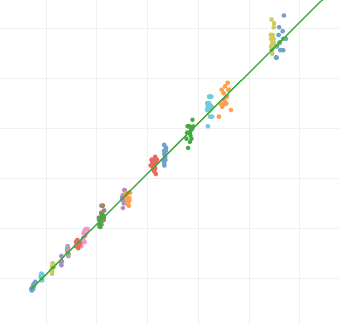
Last edited:
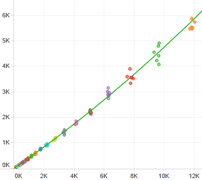
Last edited:
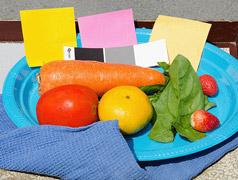
Last edited:
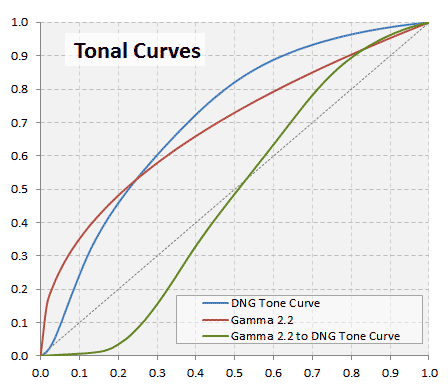
Last edited: